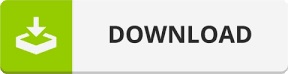
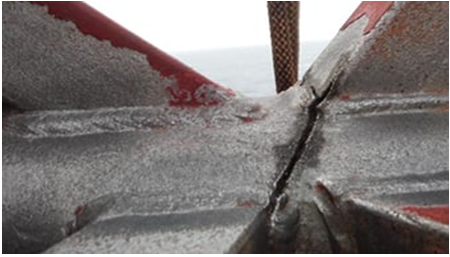
The need to bridge models of the elementary processes over different length scales and time scales makes the fatigue prediction more difficult. 2 – 5 ) Despite a great deal of effort using computational simulations, 6 – 8 ) there is still a lack of models that predict dislocation structure formation and microstructurally small crack growth under cyclic loading, and it is still challenging to develop the methodology to predict the total fatigue life with the current theoretical understanding. 1 ) By integrating these models, the performance prediction can be realized. In order to capture the complicated relationship between the manufacturing conditions and the performance, it is effective to decompose the material system into four principal elements of process, structure, properties and performance and to create models for each relationship. Against such a background, many attempts have been made to develop a methodology for predicting the fatigue performances by using physical models, numerical simulation, empirical rules and machine learning. As a demand of new materials for specific applications increases and the cost of the experiments to evaluate the time-dependent performances rises, it is becoming increasingly important to reduce the number of experiments in the material development process. Structural materials are mostly used for a long time and the time-dependent performances such as fatigue, creep and corrosion are directly related to the safety of our infrastructure.
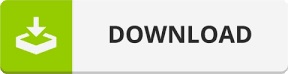